Béatrice Joyeux-Prunel argues for a post-computational framework that complements FAIR data with ethics, expertise & interpretive validation.
https://link.springer.com/article/10.1007/s42803-023-00079-6
#DigitalHumanities #Reproducibility #FAIREST #DigitalArtHistory #OpenScience
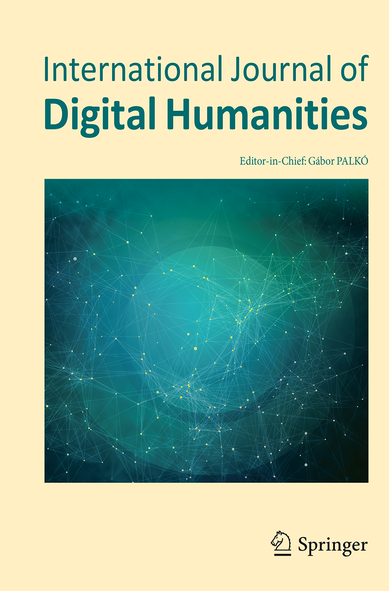
Digital humanities in the era of digital reproducibility: towards a fairest and post-computational framework - International Journal of Digital Humanities
Reproducibility has become a requirement in the hard sciences, and its adoption is gradually extending to the digital humanities. The FAIR criteria and the publication of data papers are both indicative of this trend. However, the question that arises is whether the strict prerequisites of digital reproducibility serve only to exclude digital humanities from broader humanities scholarship. Instead of adopting a binary approach, an alternative method acknowledges the unique features of the objects, inquiries, and techniques of the humanities, including digital humanities, as well as the social and historical contexts in which the concept of reproducibility has developed in the human sciences. In the first part of this paper, I propose to examine the historical and disciplinary context in which the concept of reproducibility has developed within the human sciences, and the disciplinary struggles involved in this process, especially for art history and literature studies. In the second part, I will explore the question of reproducibility through two art history research projects that utilize various computational methods. I argue that issues of corpus, method, and interpretation cannot be separated, rendering a procedural definition of reproducibility impractical. Consequently, I propose the adoption of ‘post-computational reproducibility’, which is based on FAIREST criteria as far as digital corpora are concerned (FAIR + Ethics and Expertise, Source mention + Time-Stamp), but extended to include further sources that confirm computational results with other non-computational methodologies.