Beyond just predicting words, LLMs interpolate meaning between data points, as in the recent phenomenon of AI explaining fake idioms like "you can’t lick a badger twice." The danger is that made-up "truths" will fuel future models
#AIethics #AIinEducation #AIliteracy #ChatGPT #LLM #GoogleAIOverview
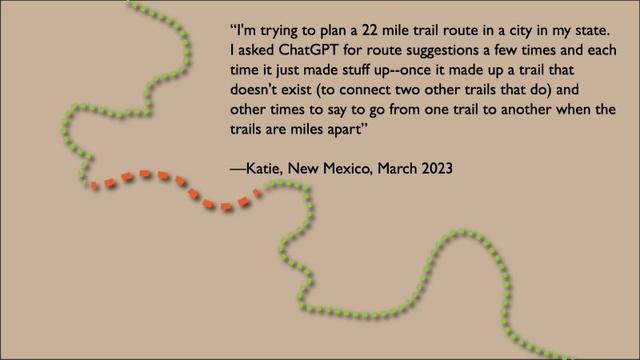
#aiethics #aiineducation #ailiteracy #chatgpt #llm #googleaioverview | Jon Ippolito
“A loose dog won’t surf?” There’s a better explanation for AI making up meanings for nonsensical idioms than just saying LLMs are word-prediction algorithms. Observers have recently been poking fun at Google’s AI Overviews for inventing meanings of nonexistent idioms like “you can’t lick a badger twice” (https://lnkd.in/e4H42Yhj). But to call this just another case of word prediction gone wrong seems to me to underestimate the power (and danger) of today’s large language models. In an abstract sense, I do believe LLMs are generating meaning, even though many of these have no correspondence with reality. One of my favorite exercises to give students at the beginning of a lesson on AI is to ask a chatbot to find the relationship between two random concepts (https://lnkd.in/exEV39HK). Some of the connections will be pretty superficial (“Taylor Swift and the Big Bang are both explosive phenomena”) while others seem more clever (“A chupacabra can appear and disappear thanks to quantum entanglement”). But all of them demonstrate a capacity to find a concept lurking in the latent space between the words in the prompt. To illustrate this dynamic visually, one of my first slides in any AI presentation usually shows the map of a trail that doesn’t exist connecting two that do, reported by a woman who prompted ChatGPT for hiking options in New Mexico. By interpolating a false trail between two real trails. ChatGPT is looking at the space between data points in its training and constructing a way to connect them. AI's tendency to find “meaning at the midpoint” is a blessing and a curse. For creative tasks, it’s why you can get LLMs to summarize the US tax code in a limerick. But it’s also why you can get answers for what researchers call false-premise questions. The average of two facts isn’t necessarily a fact. As AI-interpolated meanings continue to pop up on the Internet, they will make their way into the next generation of training data. And that's only going to make invented meanings more prevalent in the output of future models. #AIethics #AIinEducation #AIliteracy #ChatGPT #LLM #GoogleAIOverview